When we started Longtail I was a bit of an outsider. The rest of the team were real airline guys with years of experience across divisions and functions that I hadn’t even been aware of. As CTO it was my job to build the platform along with bringing the tech talent to Longtail, and my time in engineering at companies like Meta, Netflix and Expedia had shown me that it’s quite possible to use big data to optimize even the most complex problems.
Why has nobody solved this before?
My first thought was ‘why has nobody solved this problem before? Afterall, airlines have been pricing millions of O&Ds for decades. Yet the ‘longtail’ of many small, but collectively valuable routings remained stubbornly difficult to manage, as we showed in our previous post.
It didn’t take too long to realize that the scale was massive – just analyzing the opportunity-set for one client airline churned out 780 million possible itineraries when they combined their own network with those of their partners. That’s before you’ve started to think about the fares for these itineraries, or that airlines are then making hundreds of thousands of schedule changes and over a billion pricing actions every day!
Looking through a customer lens
Unsurprisingly, our first step was to break this giant challenge down into more manageable steps. First to work out what routings an airline can sensibly compete in – something that sounds obvious but, in reality, is not part of their standard process. We realized that most carriers see the globe from the perspective of the routes that they fly. Considering all possible customer journeys in the world is like looking the other way down a telescope – very disorientating.
The breakthrough was to layer on demand signals – which O&Ds are being searched for on all of possible booking platforms? Now we had a new picture both of routings that are needed, and those that are actually possible for the airline to serve in combination with partner flying.
So we had the routings, but what about the fares? That turned out to be another complex problem to solve. Creating complex algorithms (backed with sophisticated analytical models) to generate fares was just the start of the process, those fares then needed to be calibrated against market conditions (e.g. competitor pricing) and also internal constraints (e.g. ensuring that the final amount earned by the airline was still profitable). The Longtail platform had to be intelligent enough to deal with the volume and complexity of these fare-ladders but also to rapidly create fares that are ready to file into the industry database at ATPCo.
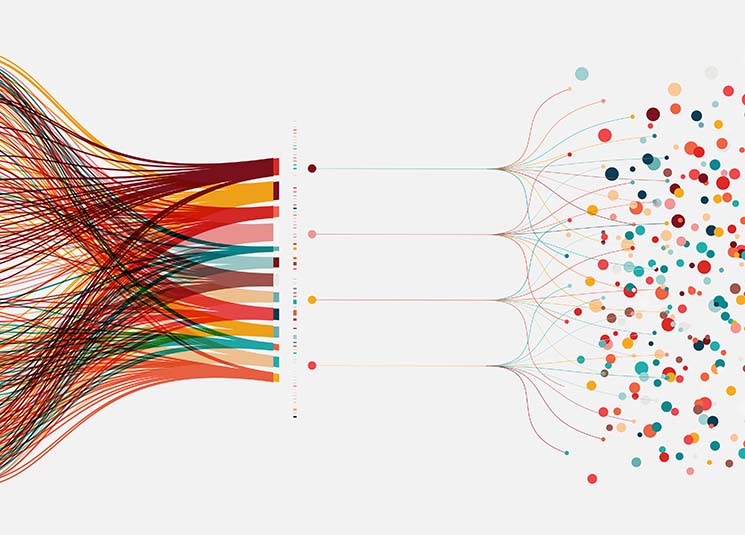
The tools to solve the problem
Just 5 years ago all of this would probably be impossible, and certainly not economically viable. But computing power has been changing rapidly and we’ve been able to take advantage of the latest in both cloud and on-prem processing to churn through oceans of data quickly and affordably.
Furthermore, we were able to architect a data layer into the Longtail platform that consumed high volume feeds from traditional industry sources like the GDS, ATPCo and OAG, alongside newer sources of demand signals and shelf availability. Building our platform on top of open-source data processing workflows ( e.g. Apache Beam), we were able to consolidate, integrate and then process these diverse data sources in Longtail’s processing and analysis layer.
So could airlines perform the same trick themselves? Well, conceptually they could. But is it worth it? The necessary combination of compute power and scarce data science skills will probably always make optimizing longtail scenarios a specialist activity. That’s why I’m proud to be part of a team who have a single-minded focus to solve this problem for airlines around the world, and to evolve the platform so it gets better and better all of the time.